Doctoral dissertation developed a machine learning model to assess collaboration quality in classrooms
Collaborative work is an important skill that schools teach children. However, if many other learnt skills can be tested with exams, the efficiency of group work is more difficult to assess. Tallinn University School of Digital Technologies doctoral student Pankaj Chejara aimed to discover behavioural patterns associated with high-quality collaboration work and develop a generalizable automated machine learning model that can assess collaboration quality in real classrooms.
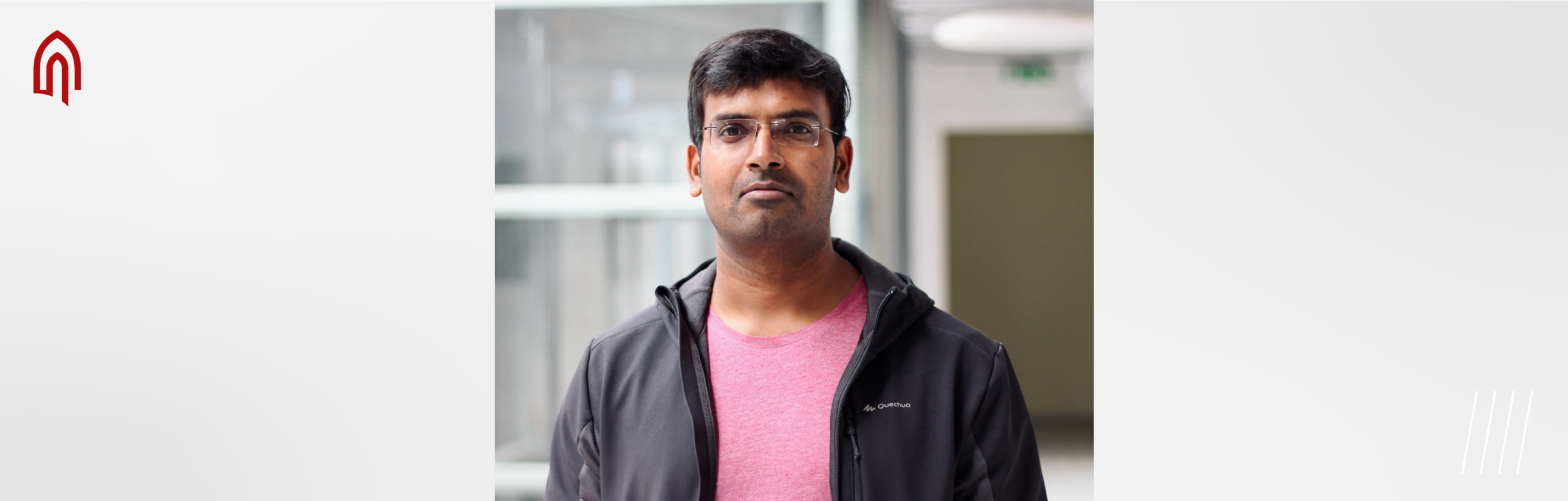
Collaboration in face-to-face settings involves participants interacting with each other through different communication channels. For example, participants convey their ideas through speech, change their tone/pitch to stress out something important or express their sentiment, complement their communication with hand gestures, and give cues of their attention with head or gaze movements. All these different types of communication channels offer substantial information to understand the quality of collaboration in a holistic way. Pankaj Chejara explored in his doctoral dissertation ways to assess the quality of collaborative work in classroom settings with the help of automated systems.
The dissertation identified several key indicators, e.g., head movement in a vertical direction resembling nodding, lip movements, speaking time, number of speaking turns, and high variation in participant’s anger levels. These key indicators were employed to automate the task of assessing the quality of collaboration in classroom collaborative learning using different machine learning algorithms. Of all tested machine learning algorithms, Random Forest consistently performed better at estimating collaboration quality, even in the case of noisy datasets. The dissertation developed models for collaboration quality estimation which were demonstrated to generalize to different schools in Estonia with only ~20% degradation in performance.
Prior research has analysed different types of communication channels and reported behavioural patterns associated with high-quality collaboration, e.g., speaking time, hand movements, and eye-gaze synchrony among participants. Chejara’s dissertation extended this further by investigating these channels in authentic classroom settings and contributed with the knowledge on how to build and assess automated systems for estimating collaboration quality in authentic classroom settings. The research contributions of the dissertations consisted of a framework to assess the generalization potential of automated systems in the education domain; a methodology to build models that can be generalized more widely; and machine learning models to assess collaboration quality. These contributions combinedly attempt to address the challenges of putting collaborative learning into practice, thus, eventually benefitting teachers to effectively monitor and support students in the development of collaboration skills.
Tallinn University School of Digital Technologies doctoral student Pankaj Chejara defended his dissertation "Classroom Collaboration Analytics: Building and Designing Automated Systems for Collaboration Monitoring in Classroom Setting" on 15 August. Dissertation supervisors were Research Fellow at the University of Valladolid Luis Pablo Prieto Santos, Associate Professor at Tallinn University María Jesús Rodríguez-Triana and Senior Researcher at the University of Valladolid Adolfo Ruiz-Calleja, opponents were Senior Lecturer at Monash University Roberto Martinez-Maldonado and Associate Professor at the University of Copenhagen Daniel Spikol.